Introduction
The Sentiment 3 Babel Machine Project uses a simple 3-label codebook with the classical negative, neutral, and positive labels, which is a consolidated version of our Emotions 6 model, where joy (4) is converted to Positive, None of Them (5) is converted to No sentiment or both positive and negative, and all other emotions are converted to Negative. While the model can classify sentences as a whole, analyzing longer sentences can yield more accurate results by breaking them down into individual clauses.
The model was prepared to make predictions on sentence-level data, meaning you should provide input that is already segmented into sentences in order to achieve optimal performance. The Sentiment Babel Machine currently uses a pooled model, that was trained on data in the following languages: Czech , English , French , German , Hungarian , Polish , Slovak , but we encourage you to also submit datasets not covered under this list, as results may be useful for additional languages due to the nature of large language models.
You can upload your datasets here for automated sentiment coding. If you wish to submit multiple datasets one after another, please wait 5-10 minutes between each of your submissions. There are two possibilities for upload: pre-coded datasets or non-coded datasets. The explanation of the form and the dataset requirement is available here.
The upload requires you to fill the following form on metadata regarding the dataset. Please upload your dataset, and in case of a pre-coded dataset, if available, please attach the codebook used beside the dataset.
The non-coded datasets should contain an id and a text column. The column names must be in row 1. You are free to add supplementary variables to the dataset beyond the compulsory ones in the columns following them.
Pre-coded datasets must contain the following columns: id, text, label. The column names must be in row 1. Uploading a pre-coded sample is optional, but it can help us with calculating performance metrics and fine-tuning the language model behind the Sentiment 3 Babel Machine. The detailed rules of validations are available here. The mandatory data format of label is numeric(integer), based on the following:
- 0: Negative
- 1: No sentiment or both positive and negative
- 2: Positive
After you upload your dataset and your file is successfully processed,
you will receive the sentiment-coded dataset and a file (in CSV format) that includes the predictions by the model.
If the files you would like to upload are larger than 1 GB, please reach out to us
with
the download
link attached (such as Dropbox or Google Drive) using our contact form.
If you have any questions or feedback regarding the Babel Machine, please let us know using our contact form. Please keep in mind that we can only get back to you on Hungarian business days.
Submit a dataset:
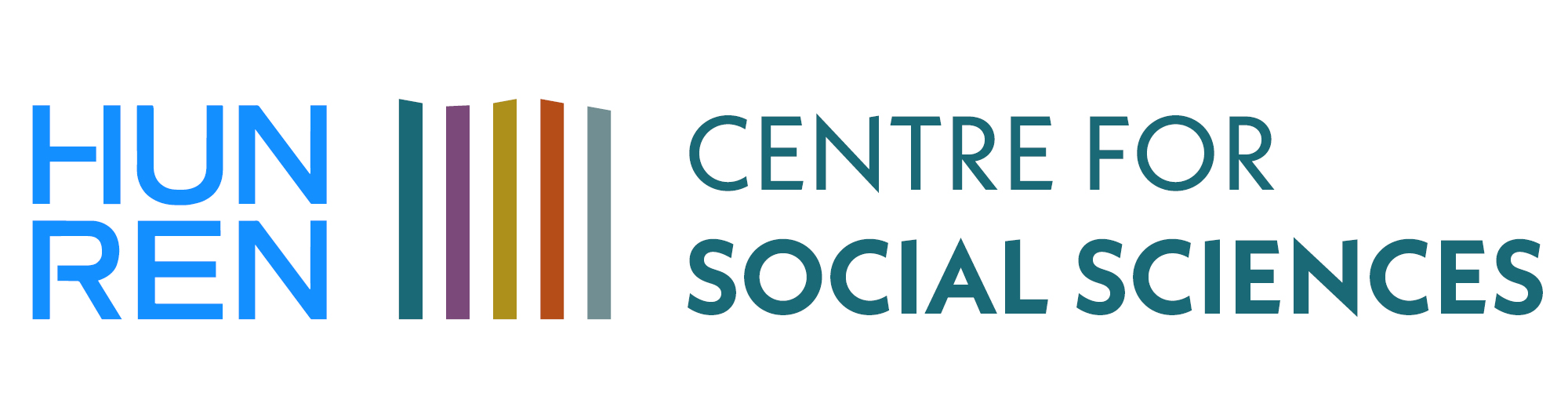
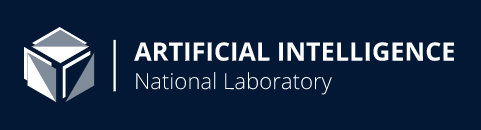
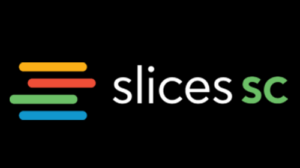
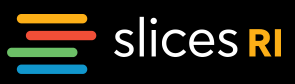

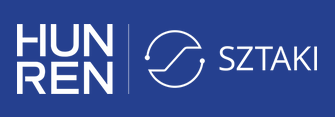
The research was supported by the Ministry of Innovation and Technology NRDI Office within the RRF-2.3.1-21-2022-00004 Artificial Intelligence National Laboratory project and received additional funding from the European Union's Horizon 2020 program under grant agreement no 101008468. We also thank the Babel Machine project and HUN-REN Cloud (Héder et al. 2022; https://science-cloud.hu) for their support. We used the machine learning service of the Slices RI infrastructure (https://www.slices-ri.eu/)
HOW TO CITE: If you use the Babel Machine for your work or research, please cite this paper:
Sebők, M., Máté, Á., Ring, O., Kovács, V., & Lehoczki, R. (2024). Leveraging Open Large Language Models for Multilingual Policy Topic Classification:
The Babel Machine Approach. Social Science Computer Review, 0(0). https://doi.org/10.1177/08944393241259434
GDPR Compliance Statement
- Nature of the Uploaded Data: The files uploaded by users to the tool do not contain personal data as defined in Article 4(1) of the GDPR, which specifies personal data as "any information relating to an identified or identifiable natural person ('data subject')".
- Data Process: The files submitted to our tool are stored in a secure cloud environment to allow processing and generation of the output (the coded CSV file). Personal data provided in connection with the file upload—such as the submitter's name, email address, and similar details—are used exclusively for the purpose of sending the coded files back to the user and identifying the organisation of our users. This processing is conducted in compliance with the purpose limitation principle (Article 5(1)(b)) and the data minimisation principle (Article 5(1)(c)) of the GDPR. By submitting the files, the user consents to this data processing, which is strictly limited to returning the results and identifying the file owner. The personal data is stored securely and retained solely for these purposes. In accordance with Article 17 of the GDPR (Right to Erasure, or "Right to be Forgotten"), users may request the deletion of their personal data at any time. Such requests will be processed promptly, and all related personal data will be permanently deleted from our systems.
- Training Purposes: We do not use personal data to train machine learning models or perform any other type of analysis. When submitting files, the submitter must declare that the uploaded CSV files do not contain any personal data, as stated in the consent agreement. This approach aligns with the purpose limitation principle (Article 5(1)(b)) of the GDPR, which requires data to be collected for "specified, explicit, and legitimate purposes" and not further processed in a manner incompatible with those purposes.
- Google Cloud Platform Compliance: The files submitted to our tool are stored in a secure cloud environment provided by Google Cloud Platform, with configurations ensuring that all processing occurs on servers located within the European Union (EU). This guarantees compliance with GDPR requirements related to data residency and cross-border data transfers. The use of Google Cloud Platform as our processing environment ensures high levels of data security and compliance with GDPR, including the application of the Standard Contractual Clauses (SCCs) for any necessary data transfers. Google Cloud's infrastructure is certified under internationally recognised standards, such as ISO 27001, ISO 27017, and ISO 27018, further ensuring the security and confidentiality of uploaded data.